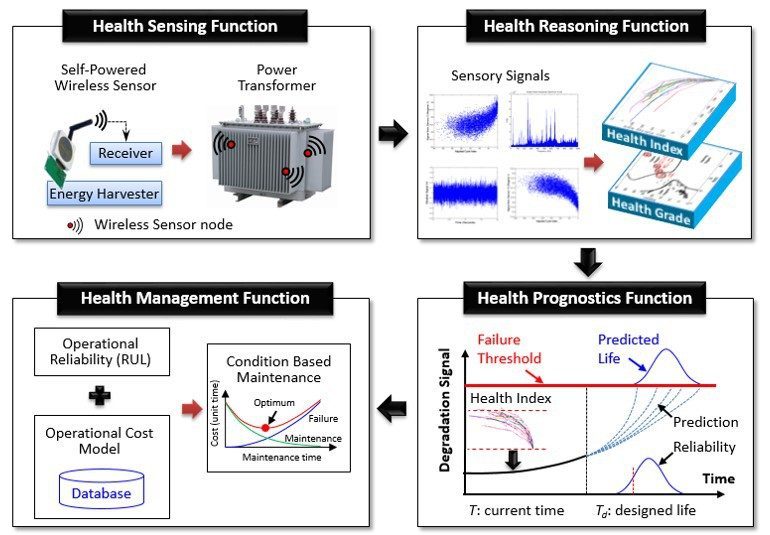
Traditional reliability analysis considers population characteristics in reliability analysis by modeling a life distribution of historical units. This traditional approach only provides an overall reliability estimate that takes the same value for the entire population. In engineering practice, we are often more interested in investigating the reliability information of a specific unit under its actual use condition in order to determine the advent of a failure and prevent the failure from occurring. Recently, prognostics and health management (PHM) has emerged as a key technology to overcome the limitations of traditional reliability analysis. PHM focuses on utilizing sensory signals acquired from an engineered system to monitor the health condition and predict the remaining useful life of the system over its lifetime. This health information provides an advance warning of potential failures and a window of opportunity for implementing measures to avert these failures. In this research area, I developed new techniques and approaches that enable optimal design of sensor networks for fault detection, effective extraction of health-relevant information from sensory signals, and robust prediction of remaining useful life.
Recent Publications
Structural Health Monitoring
- Nelson M., Barzegar V., Laflamme S., Hu C., Downey, A., Bakos, J., Thelen, A., and Dodson J., “Multi-Step Ahead State Estimation with Hybrid Algorithm for High-Rate Dynamic Systems,” Accepted, Mechanical Systems and Signal Processing, 2022.
- Barzegar V.,, Laflamme S., Hu C., and Dodson J., “Ensemble of Recurrent Neural Networks with Long Short-Term Memory Cells for High-Rate Structural Health Monitoring,” Mechanical Systems and Signal Processing, v164, 108201 (15pp), 2022.
- Barzegar V., Laflamme S., Hu C., and Dodson J., “Multi-time Resolution Ensemble LSTMs for Enhanced Feature Extraction in High-Rate Time Series,” Sensors, v21, n6, 1954 (18pp), 2021.
- Downey A.,, Sadoughi M., Laflamme S., and Hu C., “Fusion of Sensor Geometry into Additive Strain Fields Measured with Sensing Skin,” Smart Materials and Structures, v27, 075033, p1–14, 2018.
- Sadoughi M., Downey A., Yan J., Hu C., and Laflamme S., “Reconstruction of Unidirectional Strain Maps via Iterative Signal Fusion for Mesoscale Structures Monitored by a Sensing Skin,” Mechanical Systems and Signal Processing, v112, p401–416, 2018.
Bearing Diagnostics and Prognostics
- Lu H., Barzegar V., Nemani V.P., Thelen A., Hu C., Laflamme S., and Zimmerman A., “Joint training of a predictor network and a generative adversarial network for time series forecasting: A case study of bearing prognostics,”, Expert Systems with Applications, v203, 117415 (pp19), 2022.
- Nemani V.P., Lu H., Thelen A., and Hu C., and Zimmerman A.., “Ensembles of Probabilistic LSTM Predictors and Correctors for Bearing Prognostics Using Industrial Standards,” Neurocomputing, v491, p575–596, 2022.
- Shen S., Lu H., Sadoughi M., Hu C., Nemani V., Thelen A., Webster K., Darr M., Kenny S., and Sidon J., “A Physics-Informed Deep Learning Approach for Bearing Fault Detection,” Engineering Applications of Artificial Intelligence, v103, 104295 (15pp), 2021.
- Sadoughi M. and Hu C., “Physics-Based Convolutional Neural Network for Fault Diagnosis of Rolling Element Bearings,” IEEE Sensors Journal, v19, n11, p4181–4192, 2019.
- Li, Jiang Y., Guo Q., Hu C., and Peng Z., “Multi-Dimensional Variational Decomposition for Bearing-Crack Detection in Wind Turbines with Large Driving-Speed Variations,” Renewable Energy, v116 (Part B), p55–73, 2018.
Ensemble Prognostics
- Nemani V.P., Thelen A., Hu C., and Daining S., “Degradation-Aware Ensemble of Diverse Learners for Remaining Useful Life Prediction,” Under Review, Journal of Mechanical Design, 2022.
- Li, Wu D., Hu C., and Terpenny J., “An Ensemble Learning-based Prognostic Approach with Degradation-Dependent Weights for Remaining Useful Life Prediction,” Reliability Engineering and System Safety, v184, p110–122, 2019.
- Hu C., Youn B.D., and Wang P., “Ensemble of Data-Driven Prognostic Algorithms for Robust Prediction of Remaining Useful Life,” Reliability Engineering and System Safety, v103, p120–135, 2012.