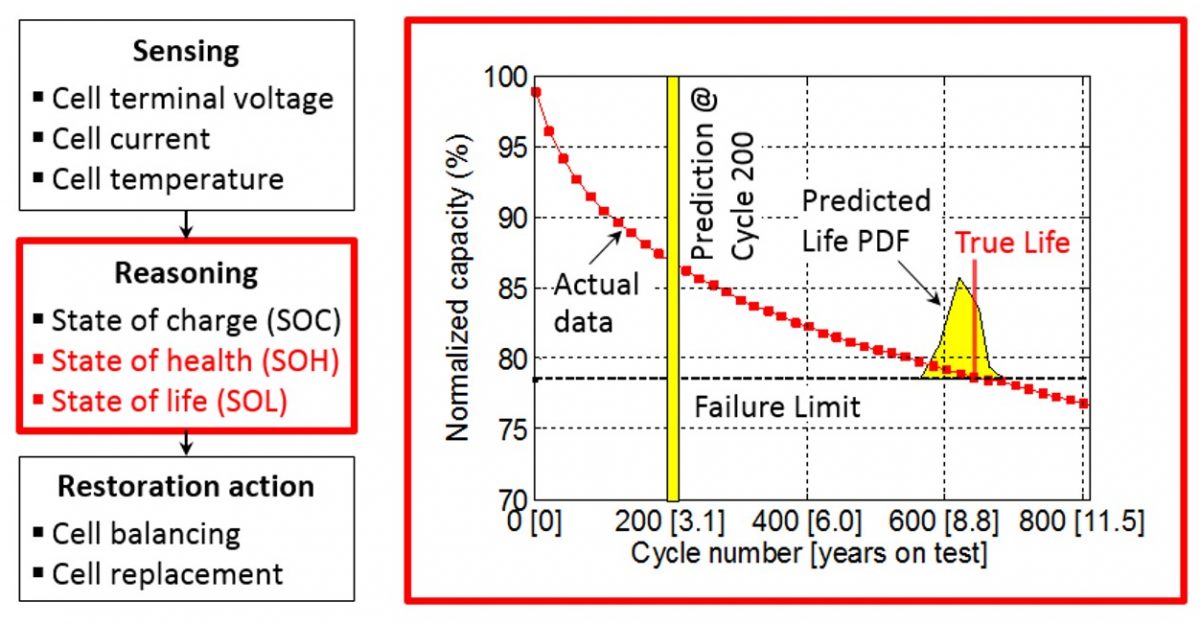
Prognostics and health management (PHM) utilizes sensory signals acquired from an engineered system to quantify the system’s health condition and predict its remaining useful life, which provides an advance warning of potential failures and a window of opportunity for implementing measures to avert these failures. To improve the reliability and safety of lithium-ion (Li-ion) batteries, it is essential to develop effective PHM techniques that can be used to detect battery anomalies in advance and prevent catastrophic failures from occurring. Boeing’s recent trouble with using Li-ion battery technology in the 787 Dreamliner planes exemplifies the cascading impact of battery failures when there is little to no way to detect abnormal degradation before a major incident occurs.
My research in this area has led to the development of a multiscale filtering method to estimate the state of health (SOH) of Li-ion batteries. This method greatly simplifies the complex process of inferring battery capacity from voltage, current, and temperature measurements, while improving prediction accuracy and efficiency. Research has also been conducted to investigate how machine learning techniques (e.g., k-nearest neighbor regression, sparse Bayesian learning, and deep belief network) can be used to analyze, in (close to) real-time, large amounts of battery measurement data for health monitoring and life prediction.
Recent Publications
- Thelen A., Li M., Hu C., Bekyarova E., Kalinin S., and Sanghadasa M.. “Augmented model-based framework for battery remaining useful life prediction,” Applied Energy, v324, 119624 (pp18), 2022.
- Thelen A., Lui Y.H., Shen S., Laflamme S., Hu S., and Hu C., “Integrating Physics-Based Modeling and Machine Learning for Degradation Diagnostics of Lithium-Ion Batteries,” Energy Storage Materials, v50, p668–695, 2022.
- Gargh P., Sarkar, A., Lui Y.H., Shen S., Hu C., Hu S., Nlebedim I.C., and Shrotriya P., “Correlating Capacity Fade with Film Resistance Loss in Fast Charging of Lithium-ion Battery,” Journal of Power Sources, v485, 229360 (7pp), 2021.
- Lui Y., Li M., Downey A., Shen S., Nemani V.P., Ye H., VanElzen C., Jain G., Hu S., Laflamme S., and Hu C., “Physics-Based Prognostics of Implantable-Grade Lithium-Ion Battery for Remaining Useful Life Prediction,” Journal of Power Sources, v485, 229327 (15pp), 2021.
- Liu J., Hu C., Kimber A., and Wang Z., “Uses, Cost-Benefit Analysis, and Markets of Energy Storage Systems for Electric Grid Applications,” Journal of Energy Storage, v32, 101731 (16pp), 2020.
- Shen S., Sadoughi M., Li M., Wang Z., and Hu C., “Deep Convolutional Neural Networks with Ensemble Learning and Transfer Learning for Capacity Estimation of Lithium-ion Batteries,” Applied Energy, v260, 114296 (14pp), 2020.
- Shen S., Sadoughi M., Chen X.Y., Hong M.Y., and Hu C., “A Deep Learning Method for Online Capacity Estimation of Lithium-Ion Batteries,” Journal of Energy Storage, v25, p100817, 2019.
- Downey A., Lui Y., Hu C., Laflamme S., and Hu S., “Physics-Based Prognostics of Lithium-Ion Battery Using Non-linear Least Squares with Dynamic Bounds,” Reliability Engineering and System Safety, v182, p1–12, 2019.
- Hu C., Hui Y., Jain G., and Schmidt C., “Remaining Useful Life Assessment of Lithium-Ion Batteries in Implantable Medical Devices,” Journal of Power Sources, v375, p118–130, 2018.